Point Prompts to improve SAMs (With table of comparison + examples)
- Denada Permatasari
- Jan 8
- 2 min read
Updated: Jan 9
In the past 6 months, the research team at Nika worked tirelessly to compare and test different Segment Anything Model (SAM) versions over spatial satellite images (widely known as GeoSAM). Dr Qiusheng Wu, a distinguished academic in GIS has done amazing work in creating this open sourced Segment-Geospatial library, accessible at https://samgeo.gishub.org/.

Now, we are very proud to announce the result of our research on their accuracy and usability. You can see the table above to read their performances at one glance and also some example outputs below.
FAST_SAM
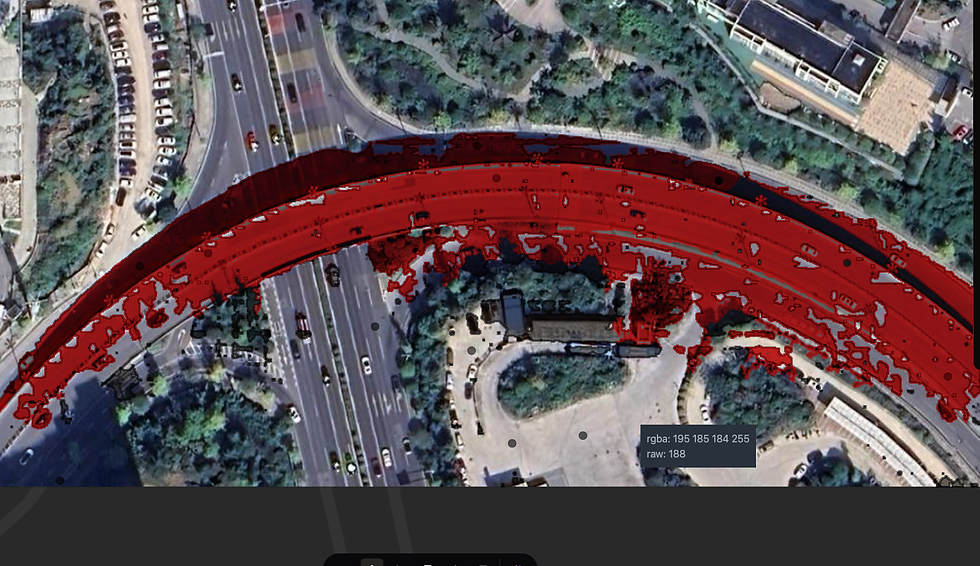
Testing the model as it is, it’s a powerful light weight model that took just a few seconds to run but had poor segmentation results with lots of holes to segmented roads.
HQ_SAM
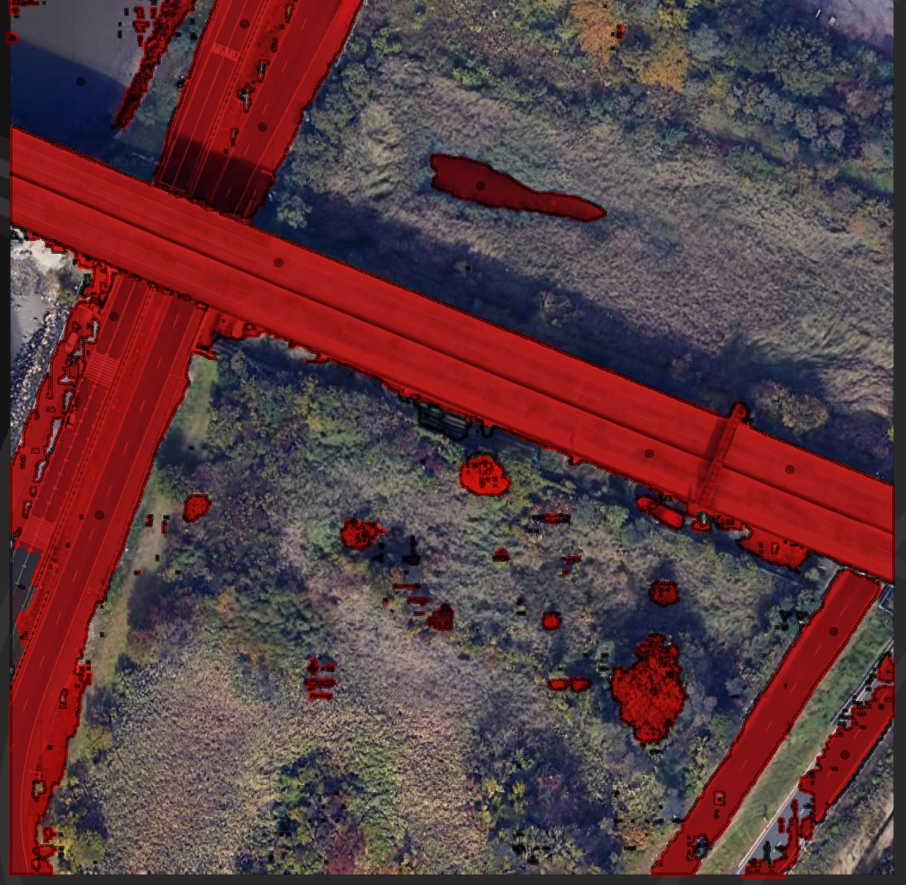
Testing the model as it is, it’s certainly more powerful but takes a longer time and still accidentally segments some trees and coastlines as roads as you can see in the image.
HQ_SAM with point prompts

Result:
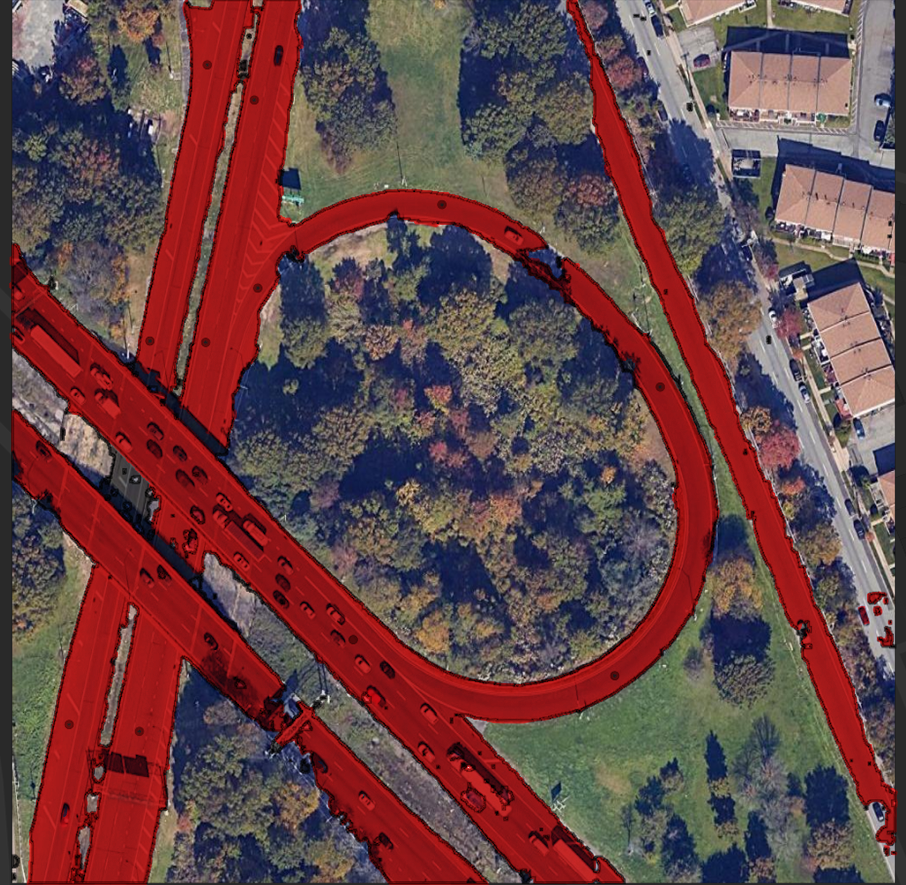
Here, we used HQ_SAM while adding in a few positive (green) and negative (red) point prompts. When we deployed it using our tech on the fly, it produced some of the best results for labeling roads.
SAMs with their precise segmentation have had a large impact on all industries since its release last year, ranging from critical segmentations of tumor cells (medical) to autonomous vehicles (transportation).
This feat has largely been possible because of GPU training on billions of images. The cost of GPUs is still very high and hence, research has been done by various people to reduce the model size without sacrificing precision the GPU based outputs give. Such fine-tuned models have been released using techniques such as Grounding DINO (zero-shot) to Few-Shot Learning (FSL).
Thanks to the techniques above, we now can segment images using high-end CPU in almost real time. This has been used in great effect for normal day-to-day images, but suffers in precision when used over satellite data which comes under its own challenges of resolution and complexity of capturing image as an “eagle-eye” view rather than conventional hand taken images.
In conclusion, we found that by using the existing models with right prompts (point segmentation), the segmentation can be achieved to a very close degree of accuracy compared with heavy GPU based models. This can be further improved with fine-tuning the out layer of the ML model to your specific segmentation scenario.
If you are someone looking to enhance GeoSAM workflow for your personal or organizational needs, let's chat! Nika has done a huge amount of work over optimizing GeoSAM over various tasks that could potentially save you time from going through the same voyage that we already charted.
Comments